I’m not a bench scientist. So before I joined BenchSci, I never thought the world needed an antibody search engine. After all, didn’t Google solve search for everything?
But now I evangelize to scientists at scale. Not only did the reproducibility crisis shock me—50% of biomedical researchers can’t reproduce their own, or someone else’s, findings—I experienced the pain of reagent failure first-hand. The time and money wasted astounds me, delaying and driving up the cost of breakthrough treatments. Antibody selection plays a pivotal role.
But since I’m not a bench scientist, I can’t personally quantify the difference between, say, searching Google Scholar and using BenchSci to find antibodies. So to help me—and you—understand this difference better, I spoke with four of our scientists, and had them show me their search process in both tools. Whether you’re a bench scientist or not, the difference may surprise you.
Google Scholar: Great with Keywords, Bad with Relevance
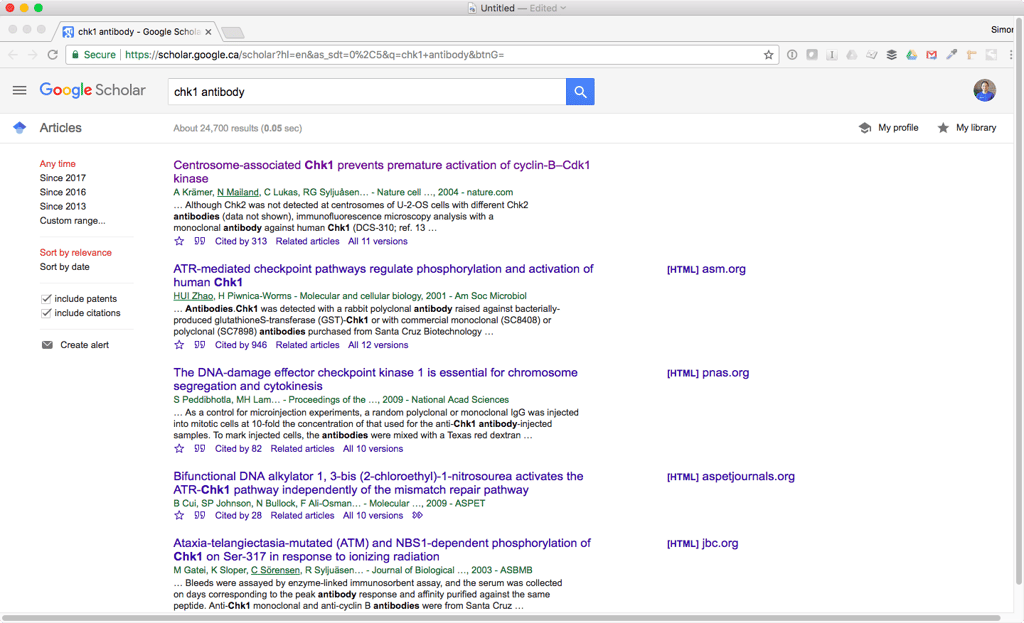
Let’s imagine you need an antibody for a new experiment or to replace a discontinued or deficient product. Or maybe you want to see results of experiments that target your protein of interest.
With Google Scholar, you would do the following:
- Go to Google Scholar
- Type in the protein
- If there’s no match, try a different name for the protein
- Scan through the results, looking at publication titles and descriptions
- Click on a result that looks promising (often opening it in a new tab)
- Make sure the researchers used the antibody in the appropriate technique (for example: western blot)
- Review the materials and methods to find the antibody vendor and SKU
- Once you find an appropriate antibody, repeat the above process several times to confirm its use in other papers
- Find the antibody on a vendor website, and purchase it
For those unfamiliar with this process, let me assure you, after following it, that it’s painful.
Searching the protein “chk1” (checkpoint kinase 1—involved in DNA repair and cell death), for example, I received 40,800 results. Some articles looked promising but lacked full text, which was a wasted click. Also, each publisher has a different user interface, forcing me to figure out several navigation structures. To help, I tried filtering results for a specific technique, such as western blot. But this was quite useless—the keyword-based search returned results if the technique were mentioned anywhere, not just in relationship to use of an antibody for chk1.
In short: Google is a great general search engine for searching keywords in websites. But Google Scholar is much less useful for searching very specific topics in scientific papers.
BenchSci: Figures and Products with Citations in 10 Seconds
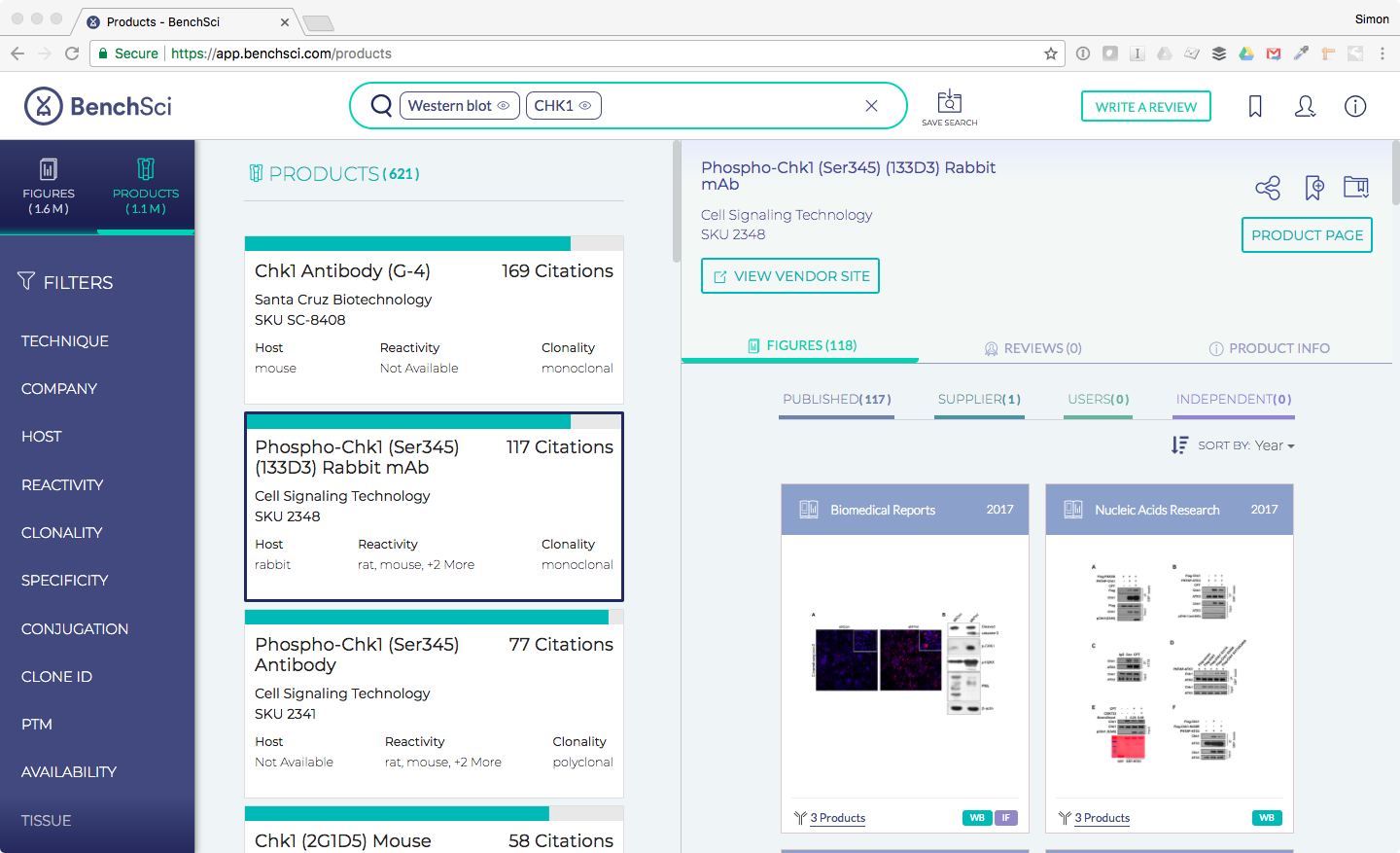
Now let’s try the same search on BenchSci:
- Go to BenchSci
- Type in the protein
- Filter by technique (as well as host, reactivity, clonality, and more)
- View figures and products with citations, and more
- Click from products to vendor product pages to purchase
This process is dramatically simpler and faster. It took me 10 seconds to find 1,231 figures and 621 products for chk1 used in a western blot. It took about 10 more seconds to choose a top-cited antibody product and click to the vendor.
Why? Because rather than scan thousands of search results, then read research papers to find antibody-related figures and products, I can scan results that are figures and products, filtered exactly the way I want, ranked from higher to lower citation count.
And since BenchSci uses machine learning to decode papers and protein-specific data tables to organize results—rather than keyword searches—these results are both more comprehensive and more relevant. For example, BenchSci incorporates aliases for protein names, so will return results for a target protein regardless of which name you use for it. Google Scholar only matches the specific name you enter.
Built for Scientists by Scientists—and Constantly Improving
And one more thing.
While I’m not a scientist, many of my colleagues—and many of BenchSci’s founders—are. And we all work closely with our users and customers to continuously improve BenchSci and tailor it to the specific needs of biomedical researchers. Google Scholar is a wonderful resource, but in serving so many scientific disciplines, it can’t serve any one perfectly.
On the other hand, we’re constantly looking to add and modify features that meet the specific needs of biomedical researchers. Some things we’re thinking about adding include:
- Price (if two products work equally well, a lab would likely purchase the cheaper one)
- Antibody features (azide-free, serum versus purified, etc.)
- Kits that combine antibodies for all proteins in a pathway
- Additional products and content sources
- Non-commercial sources of antibodies
- Differentiating whether antibodies were used in native tissues versus cell lines in an experiment
If any of these sound interesting to you, let us know in the comments. The reason we can confidently claim to be better than Google Scholar is that we designed and built BenchSci in close consultation with biomedical research scientists—including our internal team. Want to see how? Read on to learn how BenchSci works differently than Google Scholar for antibody search.