Advances in protein and antibody engineering are transforming how biologics are made. Computational biology, in particular, plays a crucial role in the design of therapeutic antibodies and in predicting target binding.
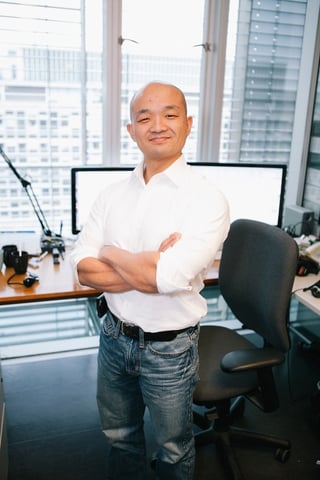
Before we head to San Diego for PepTalk 2018 (come visit us at booth #121!), we want to showcase some of the computational biology research in antibody engineering being done here in Toronto.
Accordingly, we had the great pleasure of sitting down with Dr. Philip Kim, an Associate Professor at the University of Toronto and the keynote speaker for the "Enhancing Antibody Binding and Specificity" session at PepTalk 2018.
Read on to learn more about Dr. Kim, his research, his views on machine learning in biomedical research, and his advice for young scientists in computational biology.
"Something I push very strongly in my lab is that you have to integrate computational studies"
BenchSci: Can you tell our readers your journey from being a graduate student to being an invited speaker today?
Dr. Kim: I did my grad study at Massachusetts Institute of Technology from 1998 to 2002 in physical chemistry with Dr. Bruce Tidor. The story that I always like to tell is that in the 90s it was like a hay day in computational drug development or rational drug design. When I started out in grad school the bubble was just popping. Back then it was recognized that proteins are much more complicated than what we had known, and the methods that we used to study them simply weren't good enough. So in grad school I started out doing protein modelling, but after a while I decided to focus instead on network graphs and big data, which I continued during my post-doc at Yale University, because system biology was gaining popularity at the time.
However, I always kept a structural viewpoint throughout these years as I'm a physicist by training. When I came to the University of Toronto in 2009, I moved more and more into structural studies. Something I push very strongly in my lab is that you have to integrate computational studies and by now my lab is almost half wet. Every model we made computationally gets tested in real proteins or antibodies.
"My approach is to computationally optimize the DNA library to generate a smaller, but more targeted, pool of antibody candidates"
BenchSci: Can you give us a sneak peek of your keynote presentation at PepTalk 2018?
Dr. Kim: I will be talking about our platform for the generation of targeted biologics that combines traditional protein design, thermodynamic integration and machine learning with high throughput screens. It is what I've been pushing for the last couple years whereby I believe it is critical to computationally design a large library with high quality antibody candidates which we can then screen.
Traditional antibody engineering for therapeutic purposes, as pushed by Greg Winter and other groups (including Dev Sidhu here at the University of Toronto), relies on first generating an enormous library of randomized DNA sequences via combinatorial DNA synthesis, followed by high-throughput screening using display technologies such as phage and yeast surface displays. Such strategy has had much success for generating antibodies that can bind to their target proteins simply due to the shear amount of candidates available, but it is more ideal for generating affinity tools for research use and not for biologics.
The nature of antibody binding needs to be much more precise (i.e. to a specific epitope) for biologics to have the desired therapeutic effects, and my approach is to computationally optimize the DNA library to generate a smaller, but more targeted, pool of antibody candidates, which can then be screened by display technologies. This approach is made available by the advance in custom DNA synthesis that allows us to synthesize DNA with desired and specified sequence. We, and other groups, have demonstrated that the computationally designed candidates have outperformed the combinatorial candidates in both in vitro and in vivo selection systems.
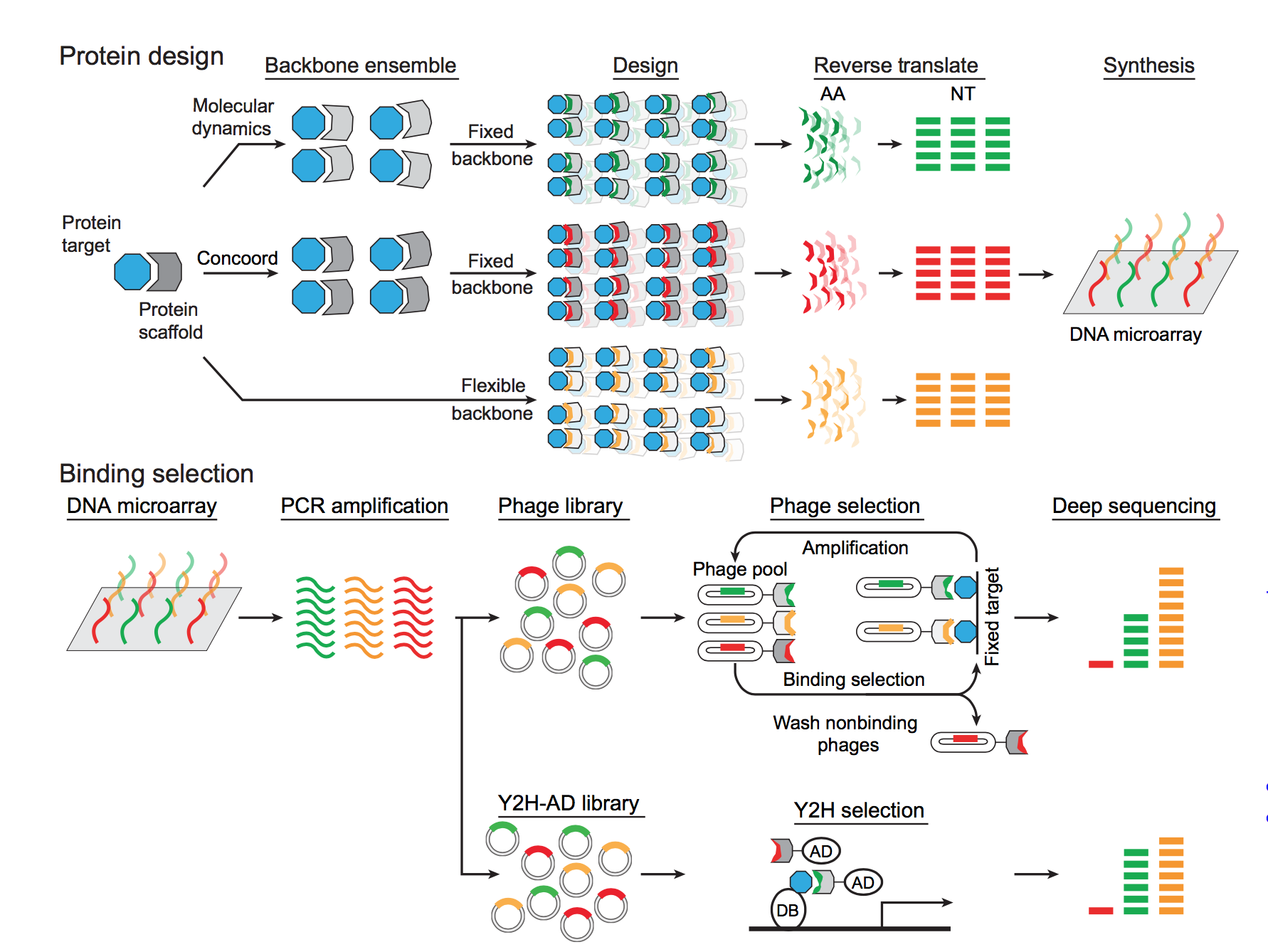
Image Credit: Sun & Seo et al., 2016 Science Advances
"Don't always chase after the mainstream but, rather, focus on your own idea"
BenchSci: What are your thoughts on the role of machine learning in biomedical research in general?
Dr. Kim: We do a bit of machine learning work with traditional energy function-based tools. I do believe there is a role for machine learning in protein structure modelling, although there hasn't yet been much success to date. There is definitely a role to play but at this point the definitive impact is still unclear.
BenchSci: What is some advice that you would like to give to the younger generation of computational biologists?
Dr. Kim: The only advice I have is that don't always chase after the mainstream but, rather, focus on your own idea. If everyone else is, for example, rushing to do large genotyping studies, and you have your own ideas, do these instead.
Dr. Kim will be giving his keynote presentation, titled "Integrated Computational Design and Experimental Selection Leads to Custom Targeted Biologics" at PepTalk 2018 in San Diego. Be sure to attend his talk if you would like to learn more about his platform for biologics design.
For more information about Dr. Kim and his current research, please visit his lab webpage.