In 2015, Nature published two commentaries that emphasized the role of antibodies in research irreproducibility (Baker, 2015; Bradbury & Plückthun, 2015). Having experienced this firsthand, we founded BenchSci. Since then, both BenchSci and the research community have come a long way in addressing the issue. One of the people driving change is Dr. David Rimm at Yale University, who has been a strong advocate for antibody validation practices (Bordeaux et al, 2010), and was cited in the Nature commentary. We chatted with Dr. Rimm about the challenge of antibody analysis, and how BenchSci can help.
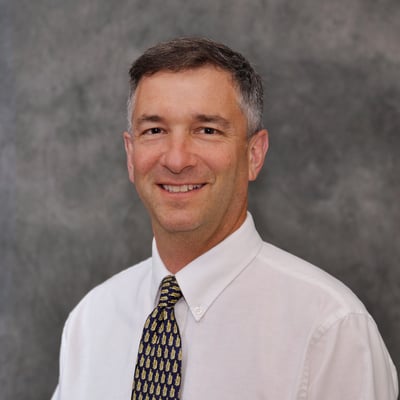
Researcher: Dr. David Rimm, Professor in Pathology and Medicine and Director of the Yale Tissue Pathology Services, Yale University
Dr. Rimm is a Professor and the Director of Tissue Pathology Services at Yale University. He completed an MD-PhD at Johns Hopkins University Medical School followed by a Pathology Residency at Yale and a Cytopathology Fellowship at the Medical College of Virginia. Dr. Rimm also serves on the CAP Immunohistochemistry committee and multiple scientific advisory boards for biotech and pharma. He is an author of over 340 peer-reviewed papers and 8 patents with an H-index of 86.
Research: Histological Identification and Screening of Cancer Biomarkers to Predict Treatment Response
Dr. Rimm’s lab works on the development of new quantitative approaches to pathology and their use to classify tumors by prognosis or predict response to targeted and immune therapies in cancer. His group also studies growth factor receptors and their signaling in new model systems. In addition, Dr. Rimm’s lab collaborates with industry labs to develop histological diagnostic tests for various types of cancer.
Challenge: Comprehensive Analyses on Antibodies and Published Data
Being a pathologist, the nature of his research requires Dr. Rimm to constantly look for new antibodies. Prior to BenchSci, Dr. Rimm typically started by searching through the websites of a selected few antibody vendors that he trusts. “Unfortunately, no company has an antibody catalog for the full 30,000 genes,” says Dr. Rimm. “For vendors that I’m not familiar with, I need to verify that the antibodies have been validated by other labs.” As a result, the Rimm lab turned to the published literature to find antibodies.
Although it is the standard practice, Dr. Rimm and his lab face a tremendous number of publications whenever they search for a new antibody. “We always try to find as much data as we can for an antibody, but we simply cannot be certain that our searches and analyses were comprehensive,” says Dr. Rimm. Indeed, there are over 28 million publications on PubMed, and the speed of publication doubles every 9 years. Compounded by the fact that not all papers cite detailed antibody information, the massive amount of available data makes the search laborious and time-consuming. “It usually takes me hours to find a good antibody candidate by reviewing the literature,” adds Jon Zugazagoitia, a visiting scientist in the Rimm lab. “And we often need antibodies for multiple targets per experiment”.
“Whenever we need an antibody, we start with BenchSci.” - Dr. David Rimm
Solution: Context-Specific Data Curation Driven by AI
To identify published antibody use cases from an ocean of data, machine learning is the perfect tool for the job. A well-trained algorithm can read millions of papers at a time to extract the precise information that scientists need to evaluate whether an antibody would be suitable for their experiments, with an accuracy that is similar to, or at times better than, that of a human scientist. “What I appreciate the most on BenchSci is the published figures,” says Dr. Rimm. “It saves me a lot of time because I only need to review the most relevant data.” Adding to this point, Yuting Liu, a graduate student in the Rimm labs, says that it only takes her about 10 minutes to find a good antibody candidate on BenchSci, and that she would tests fewer antibodies per project, which yields cost-saving of thousands of dollars.
When asked how he thinks BenchSci can help with the “reproducibility crisis” discussed in the Nature commentary, Dr. Rimm pointed out that BenchSci reinforces the practice of choosing antibodies according to published evidence by making the process much easier. This creates a positive feedback loop where, over time, the literature will only contain antibodies that have been proven to work repeatedly by independent groups. “BenchSci is a right step towards a better antibody selection process,” says Dr. Rimm. “Whenever we need an antibody, we start with BenchSci.”