Since BenchSci’s official launch in July, 2017, I’ve grown used to the 10-12 hour day typically seen in startups. With less time available to me outside of work hours, I found myself becoming increasingly more reliant on e-commerce websites, particularly Amazon, due to its amazing shopping experiences.
Trained to be skeptical throughout my PhD, I didn’t jump on the e-commerce bandwagon when it came out in early 2010s, as I was concerned about transaction frauds and the uncertainty of product quality without feeling it physically. However, after trying a couple orders from Amazon, I was immediately hooked by how easy it was for me to find what I needed from a large selection of quality products, to explore and compare a related products and, most importantly, to receive my purchase within a couple of days (sometimes within 24 hours!).
I later learned that such irresistible shopping experiences are largely driven by advances in AI technologies. As our work at BenchSci also leverages AI to help scientists find the best antibodies, I started reflecting how, back in the lab, buying biological reagents such as antibodies and siRNAs was nowhere near the same level of ease (and fun) as shopping on Amazon. I was curious what made the difference.
So I did some digging.
In this article, I will share my findings on how AI, specifically machine learning (ML), is being applied in retail e-commerce to improve shopping experiences, and how the same principles could be applied to improve the purchasing experience for biological reagents.
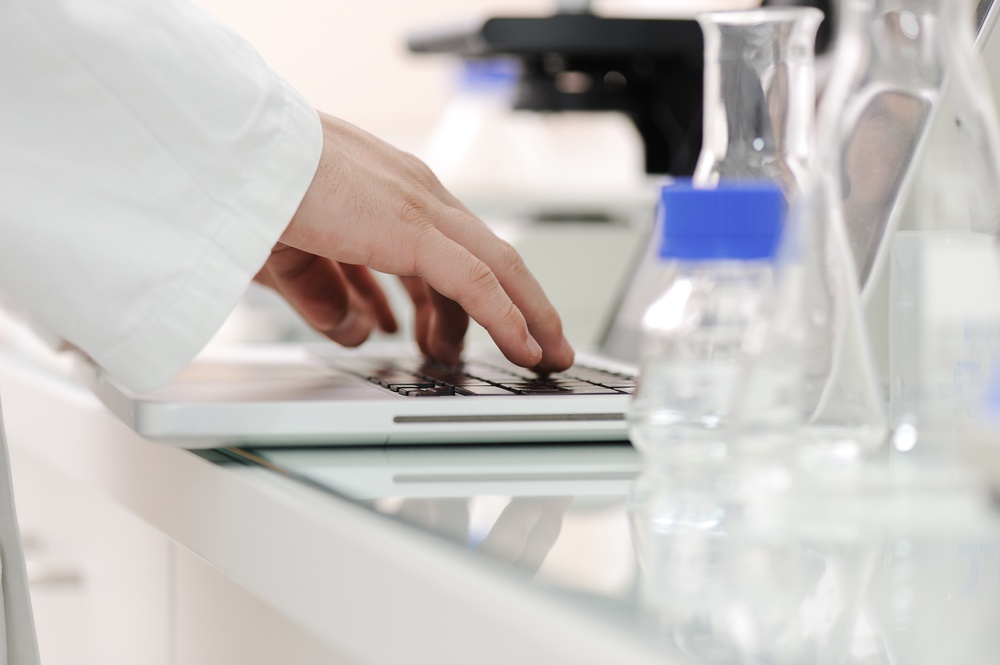
Product Recommendations to Guide Experiment Design
The respective e-commerce giants in the East and the West, Alibaba and Amazon, both leverage ML to predict what products will most likely interest customers and to provide customized recommendations based on customer searches. These ML-based recommendations drove approximately 35% of total sales at Amazon (I am part of that 35%).
While product recommendations would be equally useful for experimental design in the biological reagent market, the recommendations currently made by most vendors are based on the products that were viewed or bought together, which means those products may not necessarily be part of the same biological process (i.e. signaling pathways) or used in the same experiment. These product recommendations then, in scientists’ eyes, have little value to them, as the recommendations were not based on science but on sales.
With an ML algorithm that understands and derives the relationships between biological reagents as they were cited across the entire literature pool, however, the recommendations would be more scientifically and biologically relevant, which in turn would provide much more value to scientists and help them design their experiments based on the products that are often published together.
Reduced Shipping Time and Cost to Expedite Experiments
Since the early 2000s, one of the most prominent improvements in retail e-commerce has been the shipping time and cost. Shipping time has shortened from weeks to 1-2 days, and shipping fees range from less than $10 to sometimes free-of-charge. Such drastic improvements can be attributed to both strategically placed distribution centers and the use of ML for smart logistics. By mapping out the most efficient delivery routes through ML, Alibaba has seen 10% reduction in vehicle use and a 30 percent reduction in travel distances, which translates into faster shipping at low or no shipping fee for customers. Amazon even took a step further and started drone deliveries (yes, just like in the movies).
On the other hand, while low cost, 1-2 day shipping is slowly becoming the new norm in retail e-commerce, scientists have yet to enjoy the same perk when buying biological reagents. It is true that the shipping time has reduced to 1-2 days for them in recent years, however, the shipping cost still hovers around $30-45 per order compared to single digits for retail e-commerce. To avoid the costly shipping charges, many labs often place a bulk order until several purchasing requests have been submitted. This, in turn, delays the experiments by days to weeks and puts a burden on scientists by adding an additional layer of complexity to experiment planning.
To accelerate research discoveries, scientists should be able to receive the biological reagents they need for an experiment without unnecessary delays, and ML-guided smart logistics to reduce shipping time and cost for biological reagents would be helpful to scientists in this regard.
Demand Prediction to Enable Future Experiments
Powerful intelligence that Amazon obtains through ML is demand prediction. By analyzing a shopper’s prior on-site activity, including time on site, duration of views, links clicked and hovered over, shopping cart activity and wish lists, Amazon can predict what buyers will buy next and ensure those products are in stock in the nearest distribution center for fast and low cost-shipping.
In the biological reagent market, a common obstacle scientists face is that the desired reagent may not yet exist because they ventured into a novel research area (as they should). The lack of available biological reagents would then hinder research progress, or worse, gear scientists only towards research areas with existing reagents available.
In light of this issue, ML-driven demand prediction algorithms could be used to analyze both scientists’ purchasing history and research trends as indicated by the literature to predict and manufacture reagents ahead of time.
Let's Make Biological Reagents Like Valentine's Day Presents
Biomedical research is the cornerstone of lifesaving medicines and technologies. At the helm of this important mission, scientists should be able to obtain the reagents they need to carry out experiments as easily and seamless as possible, just like how I am able to search, compare and find the perfect Valentine’s Day gift on Amazon in less than 10 minutes and receive it the next day.
There is much room for improvement when it comes to e-commerce for biological reagents. AI technology has been shown by retail e-commerce giants to be an invaluable ally (Amazon already sells lab supplies) that could tackle challenges faced by scientists today.
What do you think? How could AI help scientists in purchasing biological reagents? Let us know your thoughts in the comment section below!